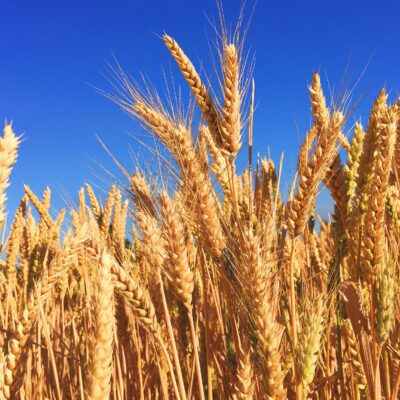
Ensuring food safety through Early Warning Detection in the AI Era
As food & beverage companies are continuously bombarded with new recalls and are faced with new and/or never-before-seen threats to their supply chain, Early Warning Detection (EWD) becomes central in their efforts to protect the health of consumers.
What is Early Warning Detection (EWD)?
Early Warning Detection refers to the practice of food & beverage companies of detecting early signals and emerging food safety risks to provide timely warnings for the implementation of mitigation measures.
The early identification of potential food safety risks enables food & beverage companies to take preventive actions before a widespread recall or outbreak occurs that could harm consumers. This approach can proactively help companies to anticipate, minimize and quickly respond to risk and health hazards as well as financial losses and impact on consumers' trust towards a brand or the food systems in general.
Critical to the successful early identification of risk and hazards in a supply chain are advanced monitoring systems, a wealth of data, a powerful data analytics tool, strategic risk assessment and rapid communication channels.
How can EWD benefit food & beverage companies?
In the recent technical report published by FAO, “Early warning tools and systems for emerging issues in food safety”, the organization discussed the utilization of both early warning & response systems (EWARs) and forecasting tools as well as, “root cause analysis” (RCA) or “environmental assessment.
Want to receive helpful food safety intelligence in your inbox?
Regarding EWARS, FAO distinguished them as:
- Risk-based predictive systems, including predictive modeling that focuses on existing knowledge of hazards that develop into risks.
- Reactive food safety-hazard-focused EWARSs that focus on recorded hazards in food products, in which FOODAKAI, the AI-powered food risk intelligence is included.
- Reactive foodborne illness (“event”)-focused EWARSs that identify irregularities in reported public health incidents.
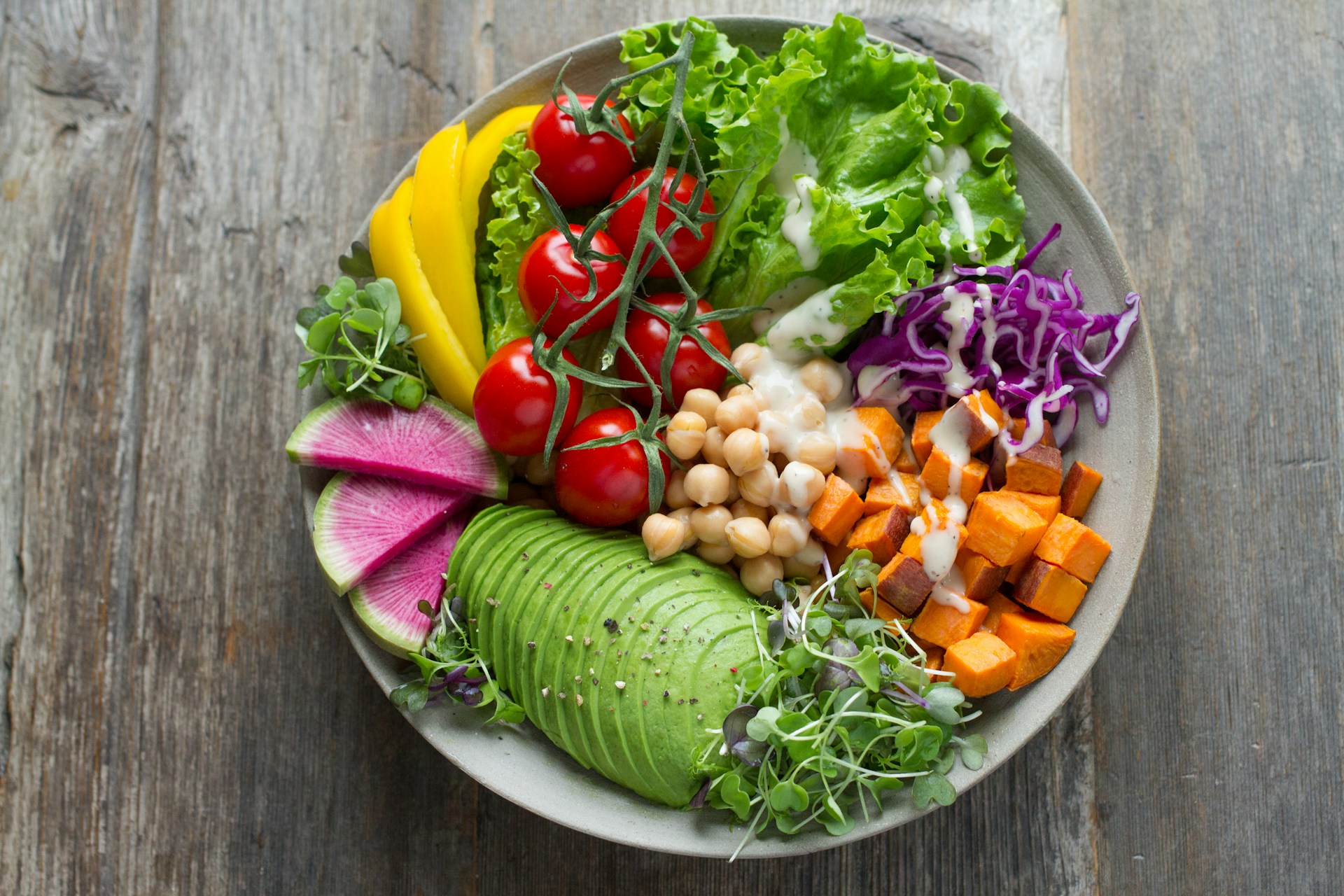
EWARSs & AI
Additionally, in the AI era, ensuring food safety has become increasingly sophisticated with the development of early warning systems that utilize advanced technologies such as classification, text mining, and time series models. These systems are designed to predict and prevent potential food safety issues before they escalate into crises.
- Classification models help in categorizing various food safety hazards based on historical data, allowing for rapid identification and response.
- Text mining techniques are employed to analyze vast amounts of unstructured data from sources such as social media, news articles, and scientific publications, extracting valuable insights and detecting emerging threats.
- Time series models, on the other hand, are instrumental in monitoring trends and patterns over time, enabling proactive measures against seasonal or recurring food safety problems.
Together, these AI-driven approaches form a robust framework for safeguarding the food supply chain, ensuring that potential risks are identified and mitigated promptly to protect public health.
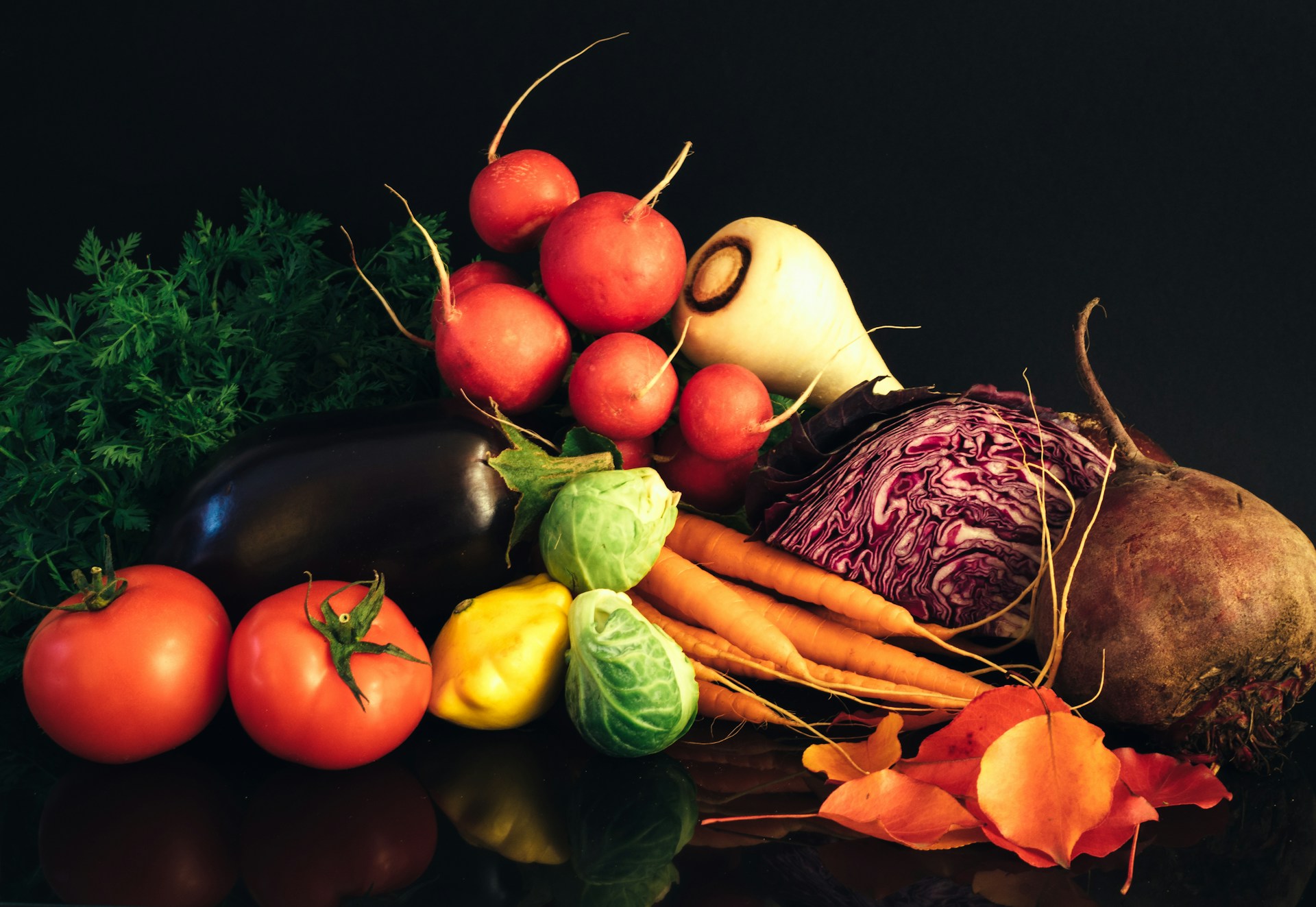
Combining EWARSs & Root Cause Analysis
“Root cause analysis” (RCA) or “environmental assessment” looks at the historic incidents to identify lessons and patterns, to prevent incidents from occurring in the future.
This combination of EWARSs and root cause analysis can help food & beverage companies formulate robust strategies that benefit from the following:
- Enhanced monitoring & assessment of risks and hazards of their supply chain by having a complete overview of the food safety history of their ingredients, products and suppliers.
- Proactive risk prevention through the early identification of emerging food safety hazards and risks for their supply chain.
- Timely identification & quick response to developing incidents, by early being notified of new recalls and taking immediate action to mitigate risks and avoid large-scale incidents
- Dynamic risk assessment of their ingredients and products based on continuously updated information on their supply chain
How does FOODAKAI help with EWD?
Food & beverage companies that are constantly faced with new risks and hazards to their supply chain, increasingly ask for a way to manage as well as anticipate future risks. FOODAKAI, the AI-powered food safety risk intelligence & forecasting platform, paves the way towards a more robust food safety risk management strategy that emphasizes proactive measures through:
- Supercharged monitoring through instant alerting on all new incidents for their supply chain and suppliers for fast incident reports through the platform's rapid communication system.
- Early identification of emerging and new risks for their ingredients through highly accurate forecasts based on continuously trained models.
- Enhanced risk assessment and hazard analysis of all ingredients highlighting the ingredients-hazard combination to adjust lab test plans accordingly.
- Streamlined supplier assessment & evaluation via food safety risk performance scoring of all sourcing partners.
- Continuous updates on the most pressing food safety risks and hazards for their supply chain.
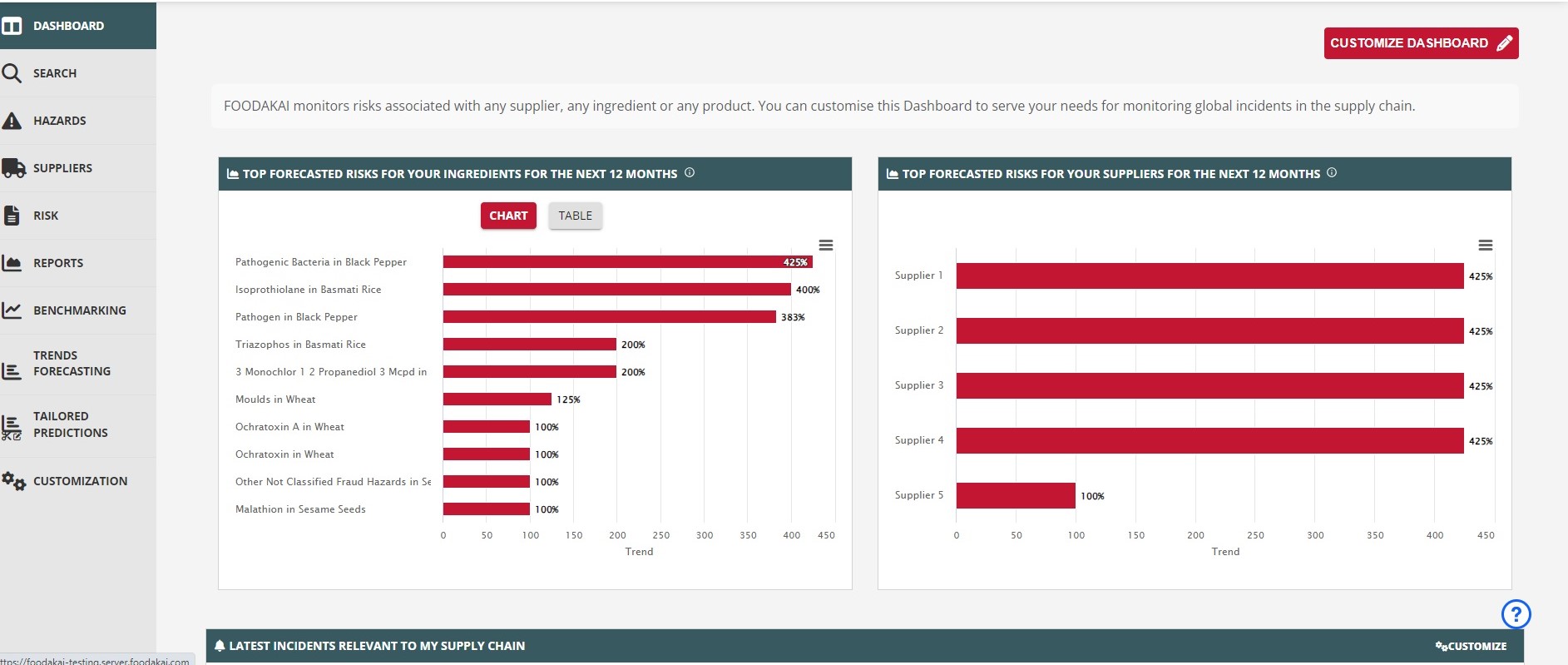
In conclusion, the implementation of Early Warning Detection (EWD) systems is critical for food and beverage companies aiming to safeguard consumer health in an era of increasing supply chain complexities and potential hazards. By leveraging advanced monitoring systems, comprehensive data analytics, and rapid communication channels, EWD enables proactive risk management and timely intervention.
The integration of AI technologies, such as classification models, text mining, and time series analysis, further enhances the predictive capabilities of these systems, allowing for the early identification and mitigation of food safety threats. Ultimately, EWD fosters a more resilient and trustworthy food supply chain, protecting both public health and brand integrity.
Experience first-hand how you can enhance early warning detection with AI in a dedicated session
Funding for this research has been provided by the European Union’s Horizon Europe research and innovation programme HOLiFOOD (Grant Agreement Number 101059813). Funded by the European Union. Views and opinions expressed are, however, those of the author(s) only and do not necessarily reflect those of the European Union or European Research Executive Agency. Neither the European Union nor the granting authority can be held responsible for them