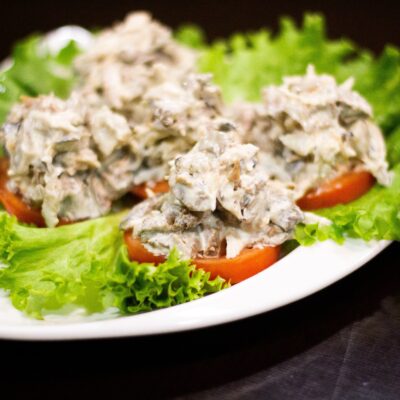
“The real power is when AI and human intelligence work hand in hand.”
Last June, season 1 of the Food & Intel Fireside Chats was concluded, with the 4th and final episode released. The podcast series where food safety professionals discuss AI and its innovative application in food risk prevention completed its inaugural season, which was hosted by Nikos Manouselis, CEO at Agroknow with guest Nicola Colombo, Global Head of SGS DIGICOMPLY.
Together they discussed the evolving role of AI in facilitating human interaction with data, especially in industries like food safety, and how AI can assist in processing vast amounts of information quickly, enhancing decision-making through improved user interfaces and real-time data analysis.
Watch the full discussion here.
The conversation also highlighted the challenges of handling large datasets, the need for significant computing power, and the importance of human oversight in tasks where precision is critical. Ultimately, AI is seen as a tool to support human expertise rather than replace it, especially in complex decision-making scenarios.
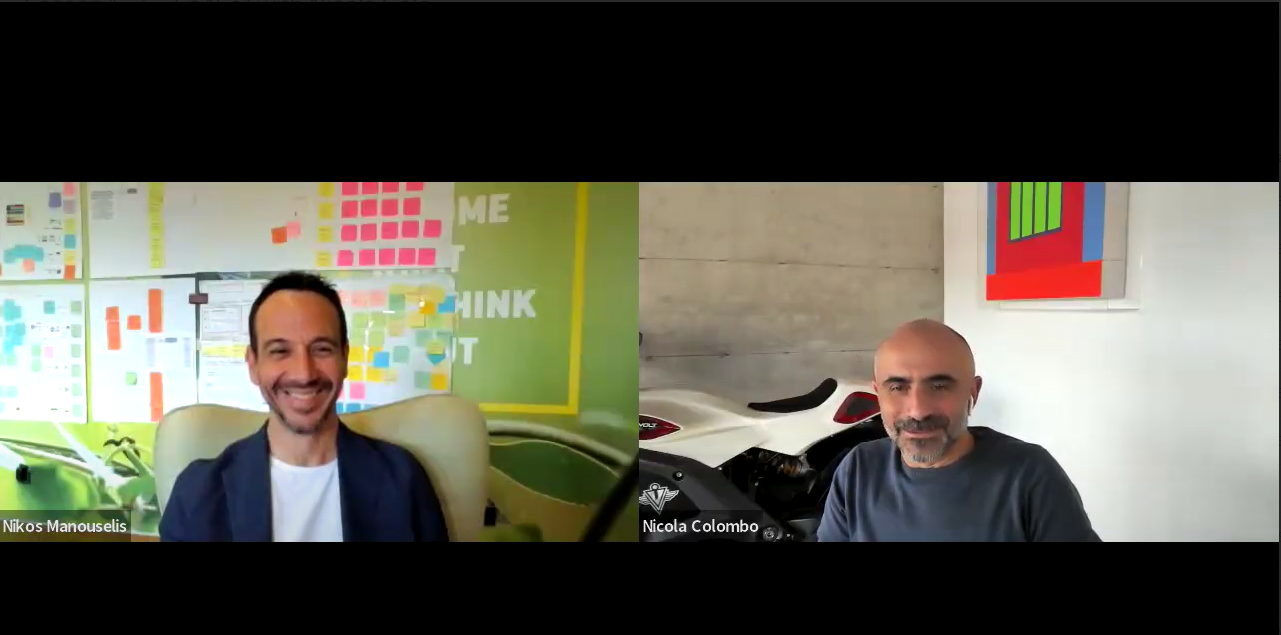
During the illuminating 40-minute episode the two delved into:
- the challenges of blending digital business within a traditional company and the importance of agility and fast-paced innovation in the data-driven world.
- the role of AI in improving data processing efficiency and supporting users by creating more dynamic, automated systems for risk assessment and regulatory compliance.
- improved user interfaces through AI: The focus is on making data retrieval and decision-making more accessible and efficient for non-experts using conversational AI and natural language interfaces.
- how large amounts of data can become overwhelming, so it's crucial to refine the interaction between AI and users to help retrieve the right information in real-time.
- the importance of real-time data and computing power: AI is evolving towards using real-time data to improve decision-making, but this demands significant computing power, which is becoming the "currency" of AI innovation.
- the food safety applications of AI as it can help with decision-making in food safety by processing vast amounts of data, with continuous human oversight.
Watch the full episode here.
Here’s a short snippet of the interview:
Nicola Colombo: So, regulations are driven by a food safety principle. So whenever there is a certain restriction on the usage of an ingredient or in the usage of a certain claim or the usage of the label, there is always a consumer safety perspective.
In the way that the product might contain certain thresholds of residues of certain chemicals from agriculture and so on so forth. So, the regulation then enter into the food safety field where normally, quality control and food safety teams in the food manufacturing or in the retail, they have to be knowledgeable about these requirements so that they can use this input to as one of the many inputs to perform their risk assessment and surveillance plan.
Risk assessment is actually part of the scope of the food safety role and the certification as well within food companies. Basically means that you need to collect these regulatory requirements, but also assess the supply chain in terms of risk and what type of and based on that generator surveillance plan.
So, the second part of our portfolio is related to food safety providing insight mostly from public available information. Transforming this raw data. Those so diversify the sources of information into a more coherent and actionable knowledge, so that again our clients can then use it as an input to understand where the risks are: if there is an emerging one, if there are some new trends, if there are some new hazards that that they need to be aware and therefore adapt their surveillance plan in a way that they somehow provide the safest possible product to their to their consumers.
Nikos Manouselis: So I hear you describing a data value chain. That is, having new updates, new types of information constantly being generated at the source and then taking lots of different steps so that eventually this can be transformed into a digital product, a data product, or a digital service to support decisions. And where does AI come to play in Nicola in this data value chain?
Nicola Colombo: Right. You, you know, you stated correctly about continuous update. So, it's a sort of pipeline now. So, once you have a starting data set and you start you know shaping it and pivoting and analysing, then you might use some data analysis tool, you may use some more programmer approach.
But when you have such a dynamic data pipeline, AI really comes into play because it allows you to perform certain tasks in all the different stages of this data processing pipeline to basically ensure you can ingest large input and distil what is relevant essentially with less effort. So, where I see AI maybe seems to be a bit trivial, but I see AI to allow more throughput with the same amount of human resources, it's that's for sure the truth.
Because we can oversee more data points, more sources and automate always with the human in the loop with the supervised approach that allows to retrieve this knowledge and supervise this data transformation process in a faster way.
Nikos Manouselis: Faster and more efficient and a wider access to all the data input that can be collected, processed, and then driven into the process. But then you're also using AI at another part of the value chain, at the end where you're supporting your users. Can describe a little bit more about how you do this?
Nicola Colombo: Yeah. Yeah. On the user side they somehow the users interact with their AI and they've been interacting with AI since we started. Now it's a little bit more on the front because there is a human to machine interaction in our system. It's mostly used from a conversational standpoint. We use more and more what is now called more like a chat interaction and it will certainly evolve to a multimodal interaction.
Also being able to use voice interaction. We've seen that even last week there was some amazing presentation from, for example, from Open AI about the new conversational capabilities. So, there's a big step up on how you can even use the voice to interact with the with machine. So those are the type of, let's say interface with AI
But the user also in a more in a more passive way, they interact with the AI because the data they see the information that they can visualise in the UI, it's basically been possible to be at that certain speed, volume, let's say and quality only thanks to the fact that behind there are AI models, AI tools, AI steps that allows to for example understanding after, you know few hours or few minutes, from data that are available on the web to get certain insight and to update certain prediction or forecasting analysis. Something that without this technology would have probably taken days or weeks before. Processing these and transforming them into an actionable insight.
Nikos Manouselis: So, practically what I hear you describing is the process of building what we would call traditionally very large knowledge base, a very large database of high-quality information. You're applying AI, advanced AI techniques and using advanced tools to populate it with high quality data in a faster, more efficient and automated way. And also to facilitate conversation, the interaction of human users with this wealth of information. So, it's easier someone to communicate with them.
Nicola Colombo: Absolutely, yeah. Absolutely. Because more data doesn't necessarily mean easier to understand. So, this large knowledge requires interaction for that retrieval that needs to be more democratised, in a way, now, even if you are not a data scientist or a data engineer or maybe a very tech savvy food engineer. You need to be able to use it. You need to generate value out of that. And so, this new kind of AI agent is certainly helping generating insights, also in an easier way in a more natural interaction.
In fact, you know, we use also the term natural language interface in AI and those are basically those text to machine, voice to machine controls and this is accelerating now because we know about this is not new, I mean everybody has an assistant on the phone since 10 years ago. I don't want to mention a brand, but I have had it for at least for 10 years. Do I use it? No, because it's not yet as fast as efficient, but it's getting there and inside my phone I have the data. So, if I want my phone to find a photo with the with the voice, I will after 5 seconds throw it away, because I say OK, it's better if I scroll the photos.
The same is happening in in any type of knowledge now any type of data set. AI is also transforming the way we can access this vast amount of. So, it's becoming more and more AI also for specialized tools, specialized knowledge fields.
Want to listen to the whole discussion?
You watch the discussion our YouTube channel here, or listen to in our Spotify account here.
Funding for this research has been provided by the European Union’s Horizon Europe research and innovation programme EFRA (Grant Agreement Number 101093026). Funded by the European Union. Views and opinions expressed are however those of the author(s) only and do not necessarily reflect those of the European Union or European Commission-EU. Neither the European Union nor the granting authority can be held responsible for them.